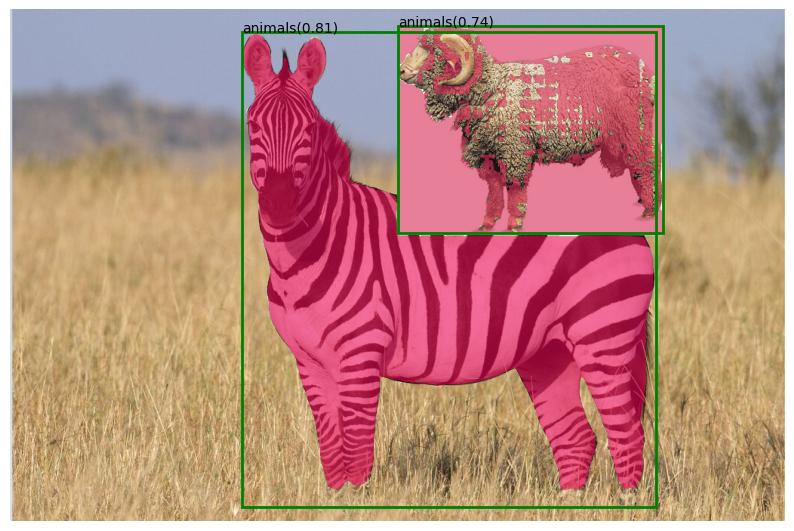
In the exciting realm of computer vision, a revolutionary technique has been transforming how machines perceive and understand the visual world around them: contextual semantic segmentation. This technique has become an essential cornerstone in the field of generative artificial intelligence, enabling a detailed understanding of images at the pixel level and paving the way for revolutionary applications across various sectors.
What is Semantic Segmentation?
Image segmentation has been a fundamental challenge in computer vision, and semantic segmentation takes it a step further. Instead of merely recognizing objects in an image, semantic segmentation assigns a label to each individual pixel of the image, resulting in a detailed and information-rich representation. This allows for precise contextual understanding of different objects present in the image.
Imagine an image where each pixel has a color corresponding to a specific category, such as red for people, blue for cars, and green for trees. This pixel-level labeling provides a comprehensive and detailed understanding of the image, which is crucial for applications like autonomous cars, robotics, agriculture, medical diagnosis, and more.
Source: The Beginner’s Guide to Semantic Segmentation
Impact on Everyday Applications
One of the most exciting applications of semantic segmentation is in autonomous vehicles. These vehicles require accurate perception of their surroundings, and semantic segmentation enables them to distinguish between pedestrians, vehicles, and other objects with high precision. Additionally, semantic segmentation is used in the retail industry to analyze store layouts and ensure proper placement of products on shelves.
In the field of medicine, semantic segmentation plays a crucial role in image-based diagnosis. Semantic segmentation algorithms help healthcare professionals identify anomalies in medical images, such as CT scans, improving diagnostic accuracy and efficiency.
Source: Datagen. CT Anomaly Classification.
Semantic segmentation is revolutionizing how brands engage with their audience. In the realm of online advertising, this technique allows for unprecedented personalization by analyzing and understanding the visual elements present in images. This leads to highly relevant ads that capture the audience’s attention and have a greater impact.
Imagine being able to display specific ads based on the objects and contexts present in the image. From the clothing a model wears to the objects surrounding them, semantic segmentation enables a seamless alignment between ads and visual content, enhancing the user experience and increasing conversion rates.
The Power of Deep Learning
Deep learning has proven to be a powerful tool in semantic segmentation. Convolutional neural networks (CNNs) have become the backbone of many successful solutions. These deep networks are trained on large datasets, automatically learning patterns and features to perform segmentation tasks with impressive accuracy.
Challenges and the Future of Semantic Segmentation
While significant progress has been made, there are still substantial challenges to address. Gathering and annotating suitable datasets for training segmentation models remains a challenging task. Additionally, the ongoing evolution of technology and demands from real-world applications continue to drive research and development in this field.
Ready to Dive into the Future of Semantic Segmentation?
At Newcom Horizons, we’re exploring how this technique is transforming how brands connect with their audience. If you’re interested in discovering how you can harness this innovation to boost your advertising strategy, feel free to reach out to us at: maddy@newcomhorizons.com. Together, we can open new doors and achieve even more exciting horizons in the realm of AI-based advertising.
By: MDesignMadrid